Understand and Evaluate Generative AI
Its rise in recent months is extraordinary primarily due to the fact that people can use natural language to prompt AI, so the use cases for it have increased multifold. Across different industries, AI generators are now being used as one’s own ‘personal assistant’ for writing, research, coding, and more.
If you’re looking to leverage these advancements in your business, now is the time to hire AI developers who can customize and deploy such solutions effectively.
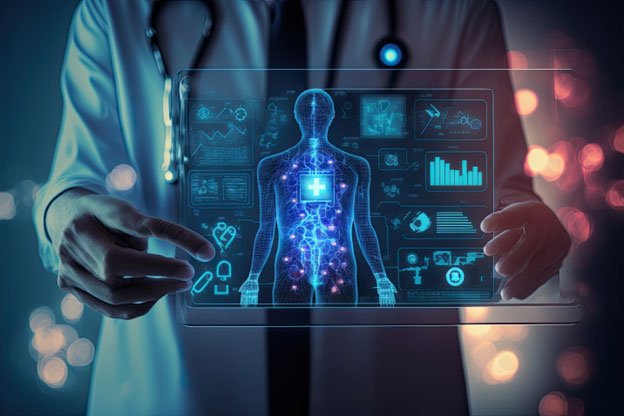
How does it work ?
Surprisingly, the technology is not as new. In 2014, generative adversarial networks, or GANs was introduced to the world — a type of machine learning algorithm – that generative AI could create new images, videos and audio of real people. Traditional AI models, known as discriminative models,could only classify or predict outcomes. For instance, a discriminative model could differentiate between images of breads and sandwiches. Whereas, generative models can produce new data, like new images of sandwiches from a huge dataset of images of sandwiches.
The basic step-by-step process for the model to work is –
- Data Collection. Specify the kind of content that the model is expected to generate
- Choose the right dataset that’s aligned with the objective
- Choose the Right Model Architecture like GANs, transformers etc
- Train the Model and refine the parameters to reduce the difference between generated output and desired result.
- Evaluate and Optimize by adjusting the model’s architecture, training parameters, or dataset
Understanding Generative AI models
Depending on the model type you’re training, GenAI models are trained a little differently. Let’s look into how the most common models are trained:
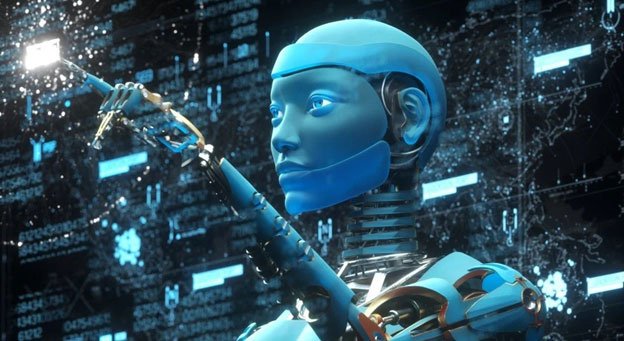
- Generative Adversarial Networks (GANs)
GANs consist of two neural networks, namely, a generator and discriminator. The generator’s job is creating new data based on existing data points, whereas the discriminator tries to distinguish between original and fake data. The generator learns to create more realistic data over time that can fool the discriminator, hence creating better quality outputs. Example – DALL-E can take a simple description in natural language and convert it into a realistic image or art
- Transformers
Transformer models consist of an encoder and a decoder. The encoder converts input text into an intermediate representation which is passed to the decoder and then converted to useful text.
Transformers,like those used in large language models (LLMs), have revolutionized natural language processing (NLP). Models like BERT and GPT are based on transformers and are capable of tasks such as text classification, text translation and text generation. LLaMA from Meta, has been trained on various data sources, including social media posts, web pages, news articles etc., to support various Meta applications such as content moderation, search and personalization.
- Diffusion models
Diffusion models learn the probability distribution of data by looking at how it diffuses throughout a system. These models destroy training data by adding noise and then learn to recover the data by reversing this noising process. Example – Stable diffusion creates photorealistic images, videos, and animations from text and image prompts
How to evaluate generative AI models
And what factors would you possibly judge them against – accuracy in the answer? simplicity in explanation? creativity in response? tone match the audience?
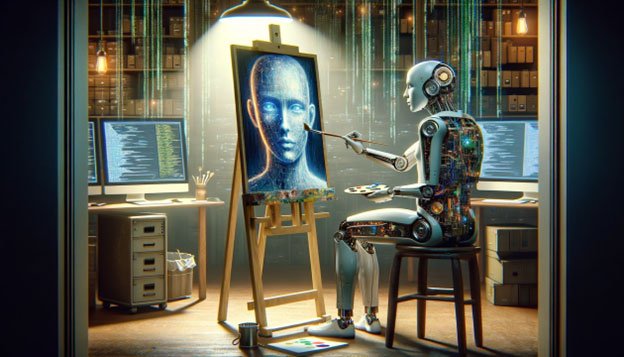
Concerns and Future State
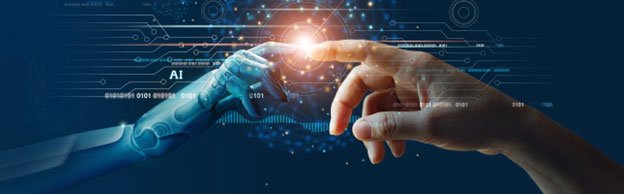
If you’re ready to embark on this journey and need expert guidance, subscribe to our newsletter for more tips and insights, or contact us at Offsoar to learn how we can help you build a scalable data analytics pipeline that drives business success. Let’s work together to turn data into actionable insights and create a brighter future for your organization.
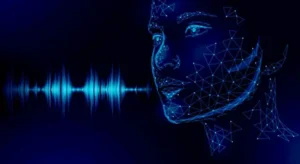
How LLMs Are Revolutionizing Text Mining and Data Extraction from Unstructured Data
Leveraging LLMs for Advanced Text Mining and Data Extraction from Unstructured Data Since digital transformation is growing exponentially, businesses generate huge amounts of unstructured data from sources like emails, PDFs,
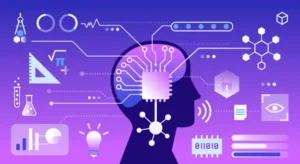
How Businesses Use LLMs for Competitive Intelligence to Stay Ahead of the Curve
How Businesses Use LLM’s for Data-Driven Competitive Intelligence to stay ahead of the curve Competitive intelligence (CI) is essential for keeping a competitive edge in today’s fast-paced business world. Businesses
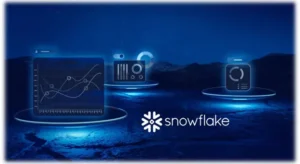
Maximizing Cost-Efficient Performance: Best Practices for Scaling Data Warehouses in Snowflake
Maximizing Cost-Efficient Performance: Best Practices for Scaling Data Warehouses in Snowflake Organizations rely on comprehensive data warehouse solutions to manage substantial volumes of data while ensuring efficiency and scalability. Snowflake,
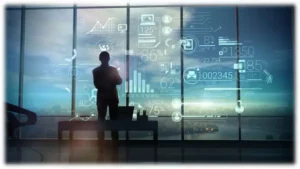
Comprehensive Guide to Implementing Effective Data Governance in Snowflake
Mastering Data Governance with Snowflake: A Comprehensive Guide Data governance is a systematic way to manage, organize, and control data assets inside an organization. This includes developing norms and policies
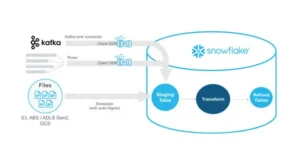
Efficiently Managing Dynamic Tables in Snowflake for Real-Time Data and Low-Latency Analytics
Managing Dynamic Tables in Snowflake: Handling Real-Time Data Updates and Low-Latency Analytics In this data-driven environment, businesses aim to use the potential of real-time information. Snowflake’s dynamic tables stand out
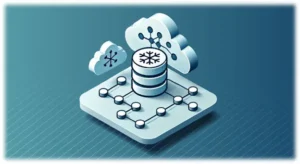
Mastering Data Lineage and Traceability in Snowflake for Better Compliance and Data Quality
Mastering Data Lineage and Traceability in Snowflake for Better Compliance and Data Quality In data-driven businesses, comprehending the source, flow, and alterations of data is essential. Data lineage is essential