Efficiently Managing Dynamic Tables in Snowflake for Real-Time Data and Low-Latency Analytics
Managing Dynamic Tables in Snowflake: Handling Real-Time Data Updates and Low-Latency Analytics
What Are Dynamic Tables in Snowflake?
- Declarative programming: Using declarative SQL, you may define pipeline results without thinking about the stages involved, decreasing complexity.
- Transparent orchestration: It allows you to easily design pipelines of various types, from linear chains to directed graphs, by chaining dynamic tables together. Snowflake handles the coordination and scheduling of pipeline refreshes depending on your data freshness goals.
- Performance benefits with incremental processing: For workloads that are well-suited to incremental processing, dynamic tables can outperform complete refreshes.
- Easy switching: With a single ALTER DYNAMIC TABLE statement, you may smoothly go from batch to streaming. You may choose how frequently data is refreshed in your pipeline, which helps to balance cost and data freshness.
- Operationalization: Dynamic tables are completely visible and controllable in Snowsight, with programmatic access to create your own observability applications.
How Dynamic Tables Work?
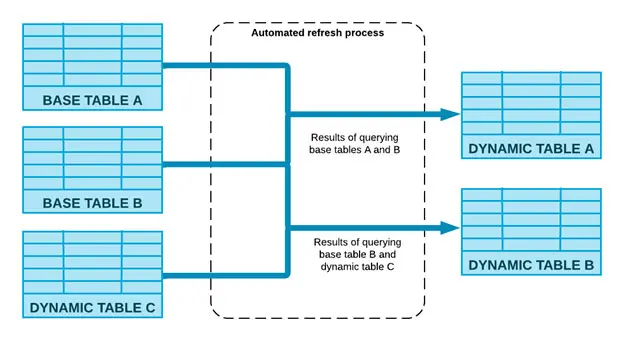
This automatic method calculates the modifications made to the base items and combines them into the dynamic table. The process does this job using computing resources associated with the dynamic table. For additional information on resources, see Understanding the cost of dynamic tables.
Challenges in Managing Dynamic Tables
- Data Consistency: Ensuring precision and preventing outdated information during frequent changes.
- Resource Consumption: Frequent changes may result in elevated computational expenses.
- Complex Query Optimization: Reconciling low-latency analytics with query efficacy.
To address these problems, a comprehensive strategy incorporating incremental updates, efficient data pipelines, and resource optimization is essential. Many organizations also collaborate with offshore analytics experts to design and monitor these strategies at scale.
Strategies for Effectively Managing Dynamic Tables
1. Incremental Data Loading
Example
The following illustrates the implementation of incremental updates in Snowflake:
-- Create a staging table for new or updated records
CREATE OR REPLACE TEMP TABLE staging_table (
id INT,
data_usage DECIMAL,
update_time TIMESTAMP
);
-- Insert new data into the staging table
INSERT INTO staging_table (id, data_usage, update_time)
VALUES (1, 1024, CURRENT_TIMESTAMP),
(2, 2048, CURRENT_TIMESTAMP);
-- Merge staging table into the dynamic table
MERGE INTO dynamic_table AS target
USING staging_table AS source
ON target.id = source.id
WHEN MATCHED THEN
UPDATE SET target.data_usage = source.data_usage, target.update_time = source.update_time
WHEN NOT MATCHED THEN
INSERT (id, data_usage, update_time)
VALUES (source.id, source.data_usage, source.update_time);
2. Optimizing MERGE Operations
Optimal Strategies for MERGE:
- Employ filters to process just pertinent records.
- Index essential columns to expedite lookups.
-- Optimized MERGE with filters
MERGE INTO dynamic_table AS target
USING staging_table AS source
ON target.id = source.id AND source.update_time > target.update_time
WHEN MATCHED THEN
UPDATE SET target.data_usage = source.data_usage
WHEN NOT MATCHED THEN
INSERT (id, data_usage, update_time)
VALUES (source.id, source.data_usage, source.update_time);
3. Partitioning and Clustering
Code Example: Clustering a Table
-- Cluster the dynamic table on frequently queried columns
ALTER TABLE dynamic_table
CLUSTER BY (update_time, id);
4. Utilizing Materialized Views for Analytical Purposes
Materialized views offer a pre-calculated result set, enhancing query efficiency for recurrent queries.
Code Example: Establishing a Materialized View
-- Create a materialized view for frequently accessed data
CREATE MATERIALIZED VIEW customer_usage_summary AS
SELECT id, SUM(data_usage) AS total_usage
FROM dynamic_table
GROUP BY id;
Best Practices for Optimizing Performance
Formulate strategies to enhance your data pipeline considering cost, data latency, and reaction time requirements.
Put the following into practice:
- Commence with a compact, static dataset to expedite query development.
- Evaluate performance with dynamic data.
- Adjust the dataset to ensure it satisfies your requirements.
- Modify your workload in accordance with the findings.
- Reiterate as necessary, prioritizing actions that have the highest performance effect.
Furthermore, downstream lag can effectively control refresh dependencies among tables, guaranteeing that refreshes occur just when required. Refer to the performance documentation for further details.
Conclusion
Using appropriate methodologies—often in partnership with offshore analytics experts—may guarantee constant, high-performance analytics, even in the most challenging real-time settings.
If you’re ready to embark on this journey and need expert guidance, subscribe to our newsletter for more tips and insights, or contact us at Offsoar to learn how we can help you build a scalable data analytics pipeline that drives business success. Let’s work together to turn data into actionable insights and create a brighter future for your organization.
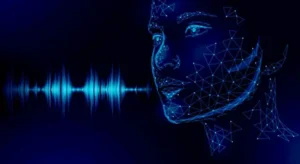
How LLMs Are Revolutionizing Text Mining and Data Extraction from Unstructured Data
Leveraging LLMs for Advanced Text Mining and Data Extraction from Unstructured Data Since digital transformation is growing exponentially, businesses generate huge amounts of unstructured data from sources like emails, PDFs,
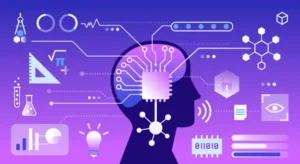
How Businesses Use LLMs for Competitive Intelligence to Stay Ahead of the Curve
How Businesses Use LLM’s for Data-Driven Competitive Intelligence to stay ahead of the curve Competitive intelligence (CI) is essential for keeping a competitive edge in today’s fast-paced business world. Businesses
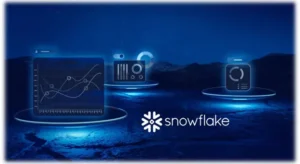
Maximizing Cost-Efficient Performance: Best Practices for Scaling Data Warehouses in Snowflake
Maximizing Cost-Efficient Performance: Best Practices for Scaling Data Warehouses in Snowflake Organizations rely on comprehensive data warehouse solutions to manage substantial volumes of data while ensuring efficiency and scalability. Snowflake,
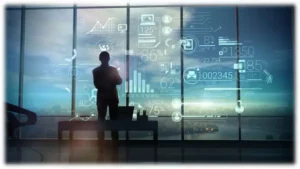
Comprehensive Guide to Implementing Effective Data Governance in Snowflake
Mastering Data Governance with Snowflake: A Comprehensive Guide Data governance is a systematic way to manage, organize, and control data assets inside an organization. This includes developing norms and policies
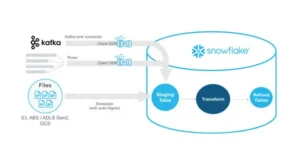
Efficiently Managing Dynamic Tables in Snowflake for Real-Time Data and Low-Latency Analytics
Managing Dynamic Tables in Snowflake: Handling Real-Time Data Updates and Low-Latency Analytics In this data-driven environment, businesses aim to use the potential of real-time information. Snowflake’s dynamic tables stand out
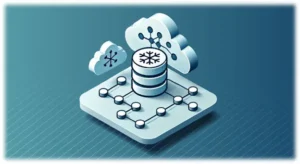
Mastering Data Lineage and Traceability in Snowflake for Better Compliance and Data Quality
Mastering Data Lineage and Traceability in Snowflake for Better Compliance and Data Quality In data-driven businesses, comprehending the source, flow, and alterations of data is essential. Data lineage is essential