Implementation of GenAI solving issues using case studies
Generative AI (Gen AI) is becoming popular across many industries, and with that, the economic opportunities it creates have become more enormous and varied. As a result, it is empowering industries to leverage data-driven insights, improve productivity, and drive innovation. Some significant sectors where Gen AI is having a tremendous impact include healthcare, pharma, and supply chain.
Streamline clinical operations
Currently, hospital providers and staff must work on multiple forms per patient, such as post-visit notes, employee shift notes, and other administrative tasks that take up hours and can contribute to hospital employee burnout. Physicians also struggle with the burdens of this administrative work.
Gen AI models can be trained on medical literature, codes, etc. so that they can effectively extract information from patient charts and discharge summaries for accurate and easy storage of medical records. They can also be used to auto-transcribe discussions and help clinicians with diagnosis. This data can be integrated into electronic health records (EHR) / electronic medical records (EMR) to maintain comprehensive patient records and treatment summaries.
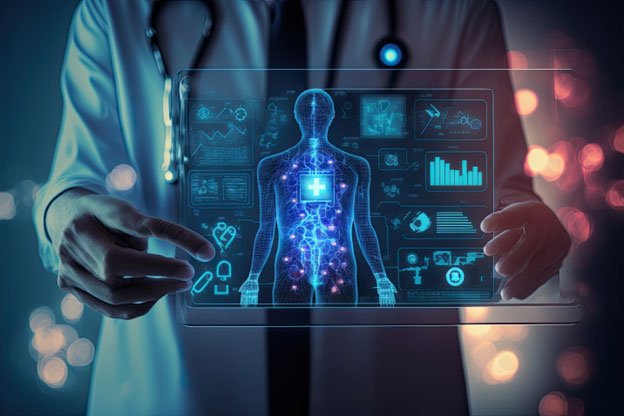
Personalized patient treatment
When fed inputs from a patient’s genetic makeup, lifestyle factors, and medical data, these Gen AI models can detect and predict how an individual will respond to different treatments but are more effective. The models are trained on large databases of patient data to identify patterns that may not be very clear for standard medical practitioners. For example, if a specific genetic marker responds exceptionally well to that medication, AI could detect helpful information like the patient’s genetics.
These models should also predict disease trajectories and the next-best treatment with a more long-term view of medical history and prognosis.
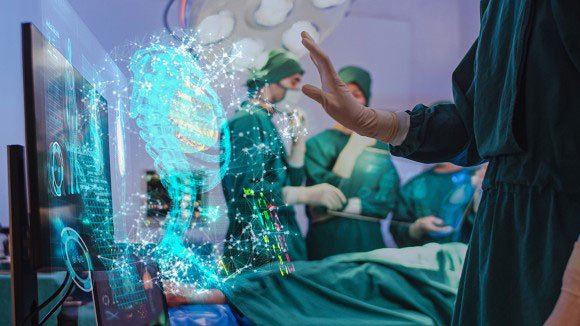
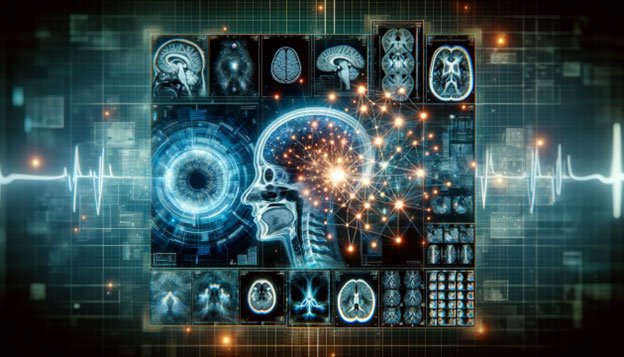
Medical Diagnosis
Large medical datasets, like MRIs and CT scans can be used to train AI models to discover patterns to help identify certain diseases. Currently, there are many instances of using GANs for image reconstruction, synthesis, segmentation and classification.
How does it work? Basically, CAD networks are used to analyze medical images and detect abnormalities like tumors, fractures, etc. Compared to traditional CAD systems, LLMs support these CAD networks by summarizing and rearranging information in natural language text format. This makes the system more accessible for patients to use and understand. LLMs can combine data from other sources inside the EHR and draw correlations between lab results, physician’s notes, and medical imaging reports to generate a more holistic view of the patient’s health.
Rapid Drug Discovery
The modern drug discovery pipeline tests millions of compounds to find those that work the best. It is a time-consuming process. AI models can speed up that process, creating the precise molecules they need in a fraction of the time. It hastens the drug discovery process as it simulates possible effects. It speeds up drug discovery by mimicking effects. We can learn much about the potential amino acid sequences and their associated structures by training these models on thousands of protein sequence-structure data. This will speed up the determination of protein structure for designing drugs or therapeutics.
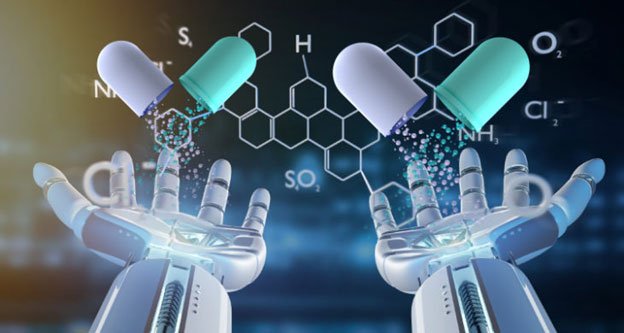
Improving logistics and distribution
AI models can also generate a contingency plan to handle disruptions like traffic bottlenecks and extreme weather. Using a mix of multiple variables like weather forecasts, vehicle specifications, traffic scenarios, fuel prices, etc., AI algorithms can determine the best transportation route. Furthermore, the AI models contribute to creating more value as they can provide textual explanations in specific directions. which could boost productivity, cut retrieval times, and optimize storage space.
In a similar way, AI-driven staffing solutions are now helping logistics companies forecast manpower needs, automate scheduling, and improve workforce utilization across regional hubs.
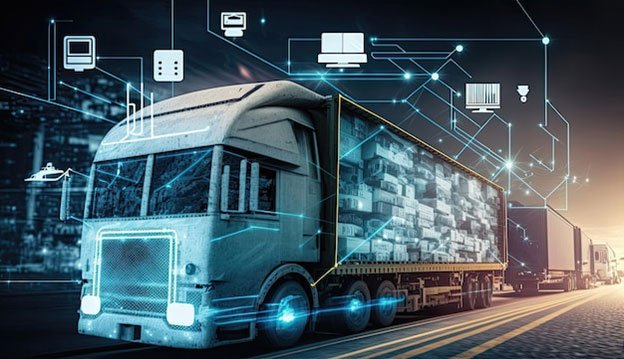
Supply chain risk mitigation
Remember when the COVID pandemic created chaos in the global supply chain? Gen AI can identify potential disruptions or risks in the supply chain based on port congestion, shipment routes, and supplier performance. This aids in arming managers with tools to prepare for disruptions before they occur.
AI models can potentially detect risks more rapidly by identifying and predicting deviations by analyzing factors like supplier performance, market data, and real-time streaming of IoT device information. AI model-based models make the supply chain more resistant to weaknesses. For example, they find obsolete logistic networks, alternative suppliers, and individual procurement methods. By reducing reliance on individual nodes within the supply chain, this proactive approach ensures that business continues despite disruptions.
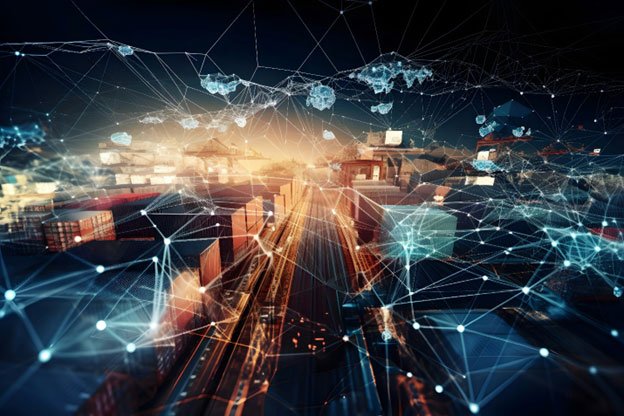
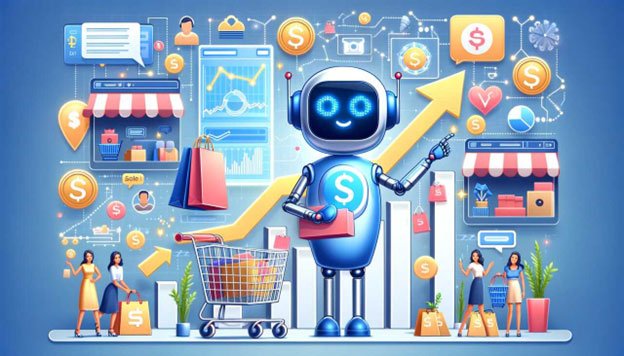
Advanced Chatbots for eCommerce & Retail
Urban Planning assistance
Gen AI can create thousands of 3D images in just a few seconds, making design processes more fluid and agile. By combining these three-dimensional images into the urban planner’s design brief and the master city plan, one can develop better approaches to urban planning. One of the more promising potentials of Gen AI is how it can simulate natural disasters like cyclones, floods, or earthquakes and tell us how robust the city’s infrastructure is. Gen AI can stimulate population increase and demographic trend dynamics to create possible new city growth models, thus predicting the level of infrastructure investment in housing, transport, or public service that supports urban living. As GenAI capabilities grow, companies are also increasingly adopting AI-driven staffing solutions to source the right AI talent for these complex implementations. This means that cities can ensure more intelligent use of resources by integrating AI into urban management systems, predicting the need for maintenance, or making their operations more streamlined. Computer models improve infrastructure maintenance and repair scheduling, reducing expensive breakdowns.
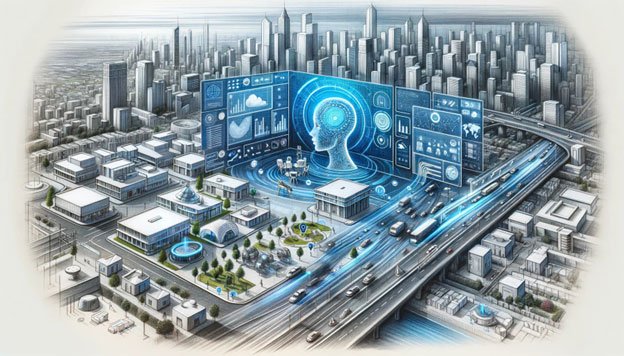
If you’re ready to embark on this journey and need expert guidance, subscribe to our newsletter for more tips and insights, or contact us at Offsoar to learn how we can help you build a scalable data analytics pipeline that drives business success. Let’s work together to turn data into actionable insights and create a brighter future for your organization.
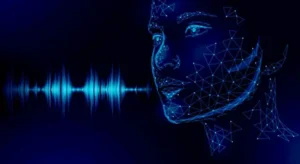
How LLMs Are Revolutionizing Text Mining and Data Extraction from Unstructured Data
Leveraging LLMs for Advanced Text Mining and Data Extraction from Unstructured Data Since digital transformation is growing exponentially, businesses generate huge amounts of unstructured data from sources like emails, PDFs,
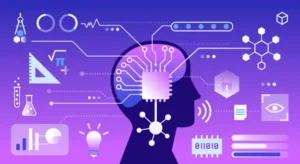
How Businesses Use LLMs for Competitive Intelligence to Stay Ahead of the Curve
How Businesses Use LLM’s for Data-Driven Competitive Intelligence to stay ahead of the curve Competitive intelligence (CI) is essential for keeping a competitive edge in today’s fast-paced business world. Businesses
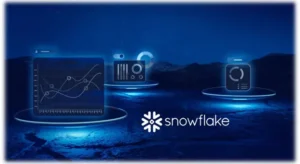
Maximizing Cost-Efficient Performance: Best Practices for Scaling Data Warehouses in Snowflake
Maximizing Cost-Efficient Performance: Best Practices for Scaling Data Warehouses in Snowflake Organizations rely on comprehensive data warehouse solutions to manage substantial volumes of data while ensuring efficiency and scalability. Snowflake,
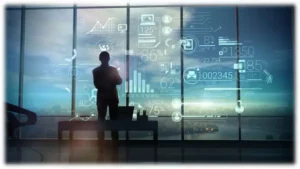
Comprehensive Guide to Implementing Effective Data Governance in Snowflake
Mastering Data Governance with Snowflake: A Comprehensive Guide Data governance is a systematic way to manage, organize, and control data assets inside an organization. This includes developing norms and policies
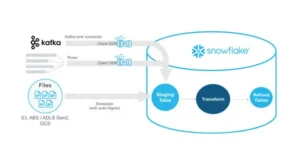
Efficiently Managing Dynamic Tables in Snowflake for Real-Time Data and Low-Latency Analytics
Managing Dynamic Tables in Snowflake: Handling Real-Time Data Updates and Low-Latency Analytics In this data-driven environment, businesses aim to use the potential of real-time information. Snowflake’s dynamic tables stand out
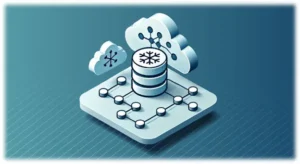
Mastering Data Lineage and Traceability in Snowflake for Better Compliance and Data Quality
Mastering Data Lineage and Traceability in Snowflake for Better Compliance and Data Quality In data-driven businesses, comprehending the source, flow, and alterations of data is essential. Data lineage is essential