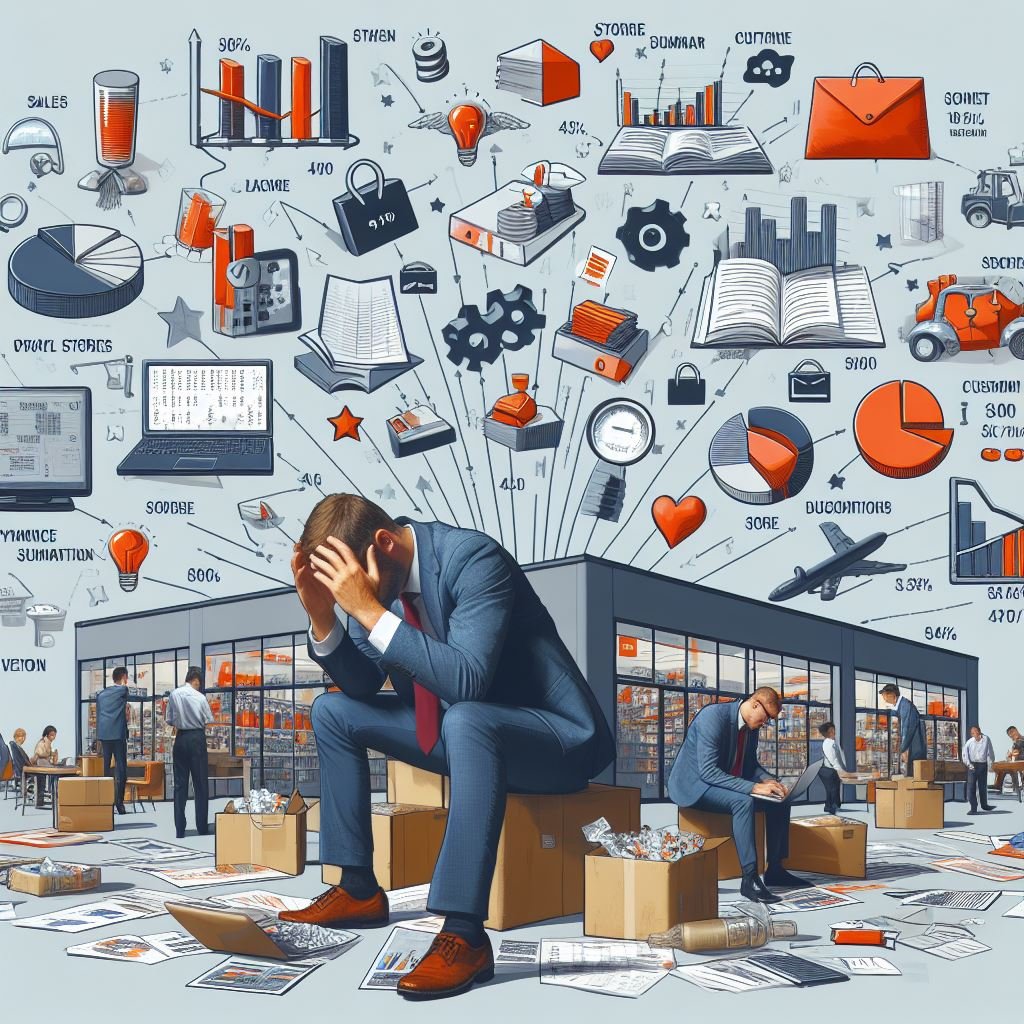
Case Study: Transforming Data Analytics for a Large Retailer
One of the largest national retailer, with hundreds of stores and a significant online presence, faced significant challenges due to scattered data from multiple sources—sales, customer feedback, inventory, and online interactions. This fragmentation made it hard for the retailer to understand their business needs and make informed decisions.
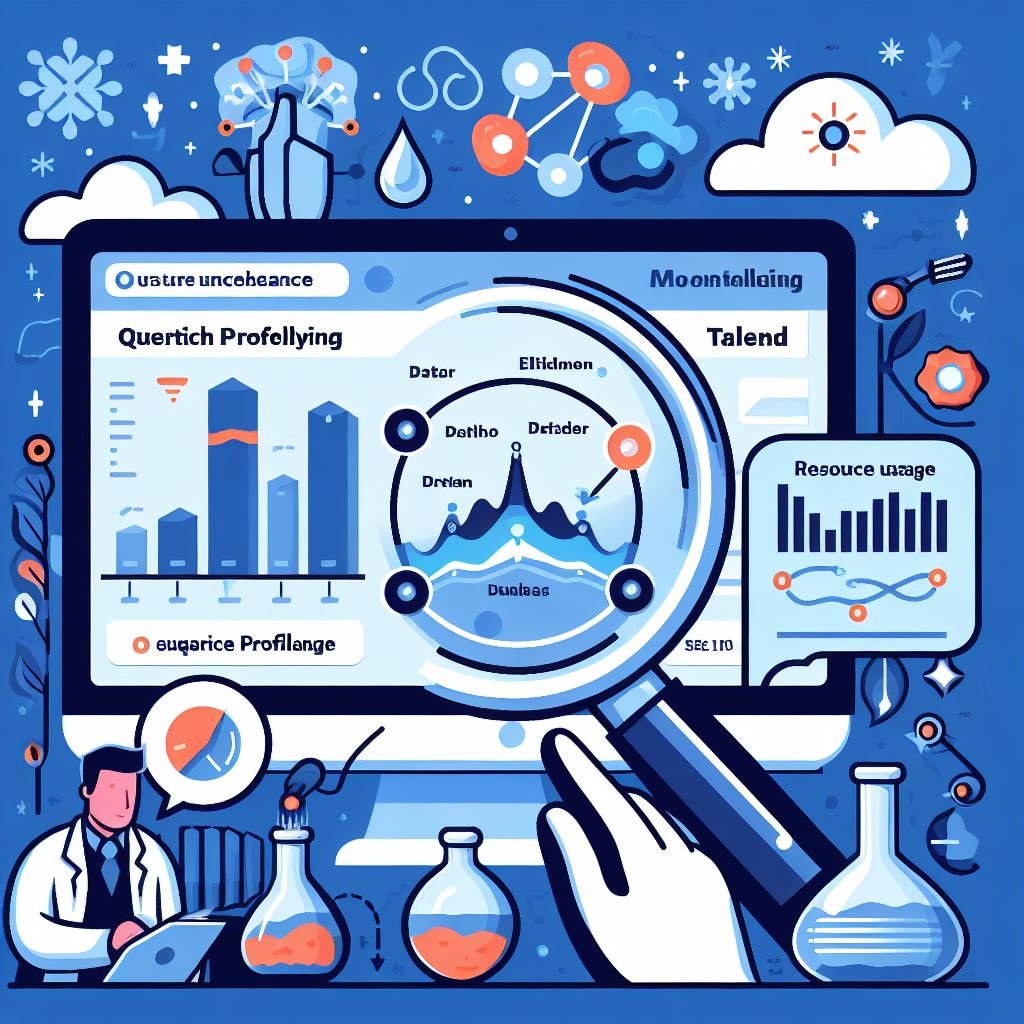
Transforming Data Analytics for a Large Retailer
Challenges
Inventory Management: The retailer needed to know when to reorder stock to avoid overstocking or running out of popular items. With data spread across multiple systems, getting a clear picture of inventory levels and sales trends was challenging.
Customer Feedback Analysis: Understanding customer feedback is crucial for improving products and services, but the data was scattered across emails, online reviews, and in-store surveys. Without centralized data, it was hard to get actionable insights.
Sales Performance Tracking: The retailer wanted to track which products were performing well in different regions and which needed additional marketing support. However, their sales data was in different databases, complicating their ability to get a unified view of performance.
Marketing Campaign Effectiveness: The retailer needed to assess the effectiveness of their marketing campaigns to understand which channels were driving the most sales and customer engagement. The data was scattered across social media, email marketing, and advertising platforms, making it hard to gauge success.
Operational Efficiency: The retailer needed to streamline store operations to reduce costs and improve customer satisfaction, but the fragmented data made it difficult to identify areas for improvement.
Due to this fragmentation, the retailer couldn’t make informed decisions quickly or efficiently. They needed a scalable data analytics solution to unify their data and stay ahead of the competition.
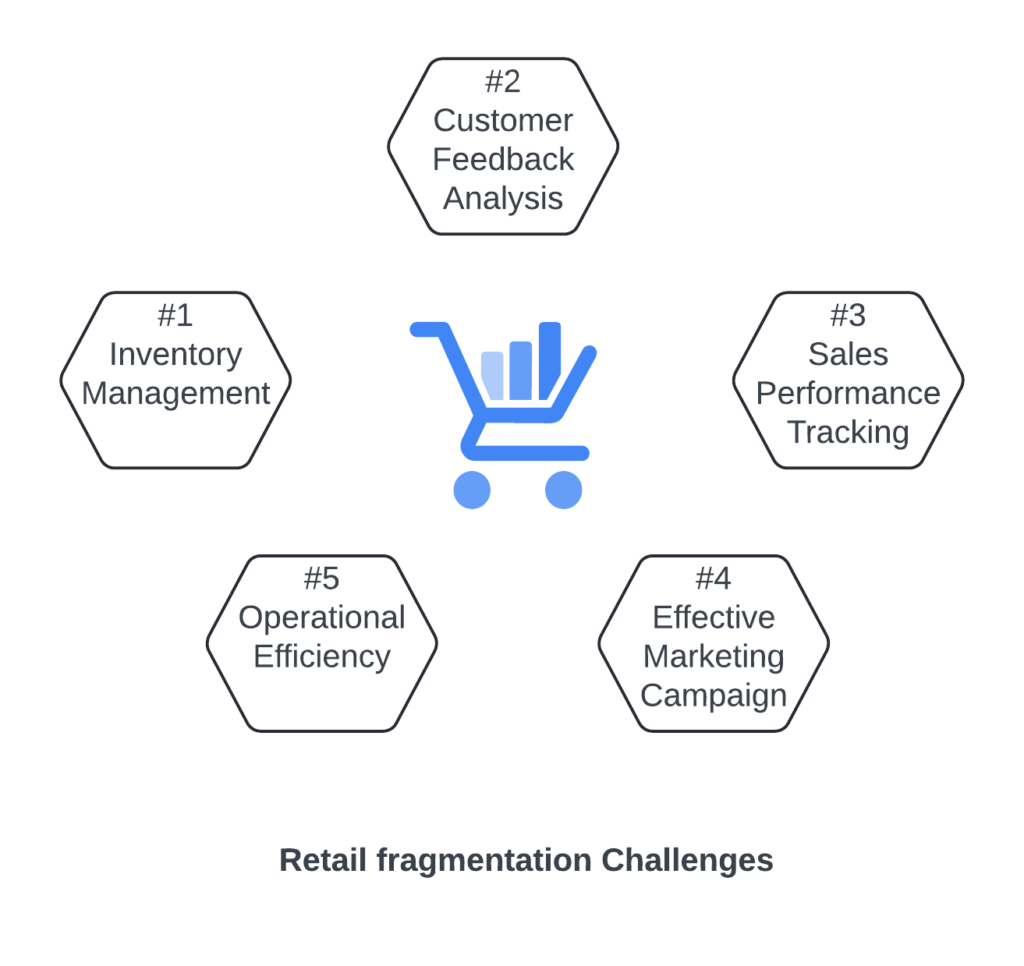
Our Solution
We partnered with the retailer to build a scalable data analytics pipeline. Using Talend for data integration and transformation and Snowflake for data warehousing, we designed a system that connected all their data sources, centralized the data in Snowflake’s cloud-based warehouse, and applied transformations to generate useful insights.
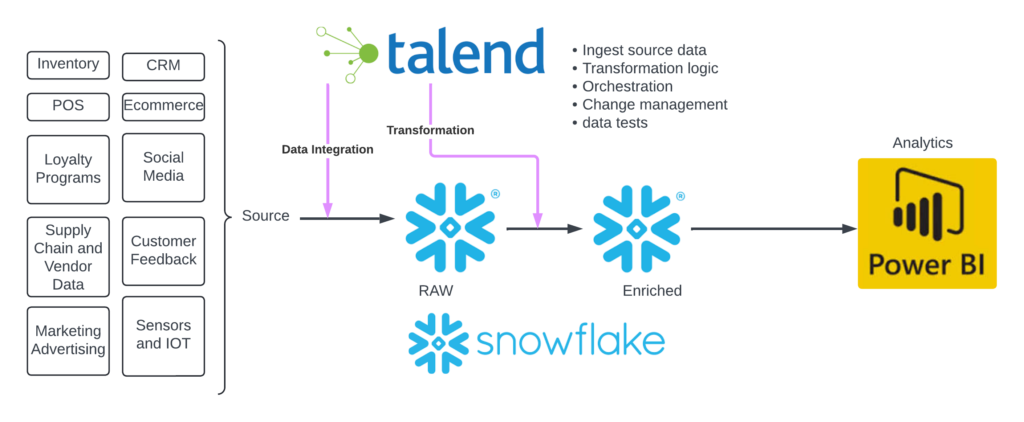
The Results
1. Integrated Data
Using Talend, we integrated data from over 10 different sources, including databases, cloud storage, and APIs. What used to take weeks to compile now takes only a few hours, saving a significant amount of time.
2. Speed and Efficiency
The new pipeline processes data 50% faster than the old system. This means the retailer can generate daily sales reports in minutes instead of hours, allowing for quicker business decisions.
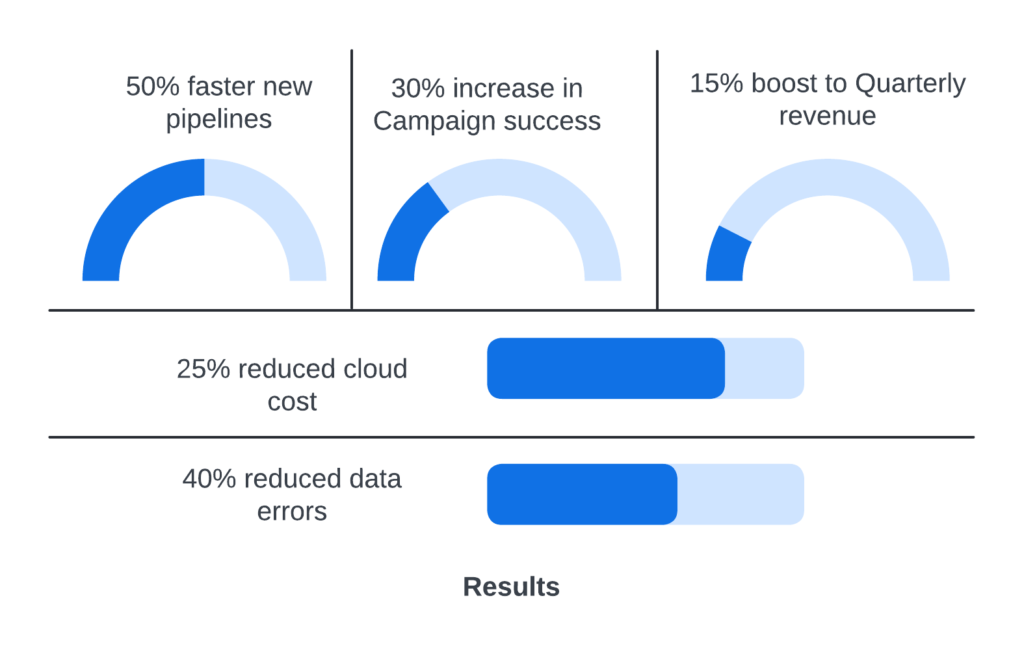
3. Better Customer Insights
With Talend’s advanced transformation features, the retailer created detailed customer profiles and ran more targeted marketing campaigns, resulting in a 30% increase in campaign success. This led to a 15% boost in quarterly revenue.
4. Cost Savings
Snowflake’s flexible scaling allowed the retailer to adjust resources as needed, reducing cloud costs by 25%. This saved money they could invest in other business areas.
5. Improved Data Quality and Compliance
The retailer reduced data errors by 40% with the new data governance framework and ensured compliance with regulations like GDPR. This minimized the risk of legal issues and enhanced customer trust.
Best Practices and Common Pitfalls
Here are some best practices to build a successful data analytics pipeline:
Data Governance: Develop a well-defined data governance strategy. Ensure all ETL/ELT processes are properly documented, and perform regular data quality checks.
Team Training: Invest in training your team to understand the pipeline’s architecture and purpose. This minimizes errors and ensures efficient operations.
Avoid common pitfalls, such as overcomplicating your pipeline design, which can lead to maintenance challenges. Also, don’t over-provision resources, as this can increase costs without adding value. Properly manage data security and compliance to avoid potential legal issues.
Conclusion
The retailer’s success story demonstrates the value of a well-designed data analytics pipeline. By integrating their data, improving processing speed, gaining better insights, and reducing costs, they could make more informed decisions and drive business growth. This case study shows that a scalable data analytics pipeline can significantly impact business success.
If you’re ready to embark on this journey and need expert guidance, subscribe to our newsletter for more tips and insights, or contact us at Offsoar to learn how we can help you build a scalable data analytics pipeline that drives business success. Let’s work together to turn data into actionable insights and create a brighter future for your organization.
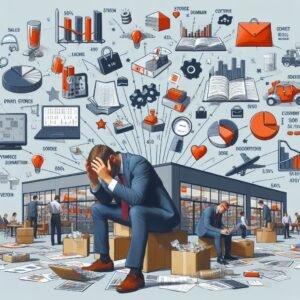
Case Study: Transforming Data Analytics for a Large Retailer
One of the largest national retailer, with hundreds of stores and a significant online presence, faced significant challenges due to scattered data from multiple sources—sales, customer feedback, inventory, and online
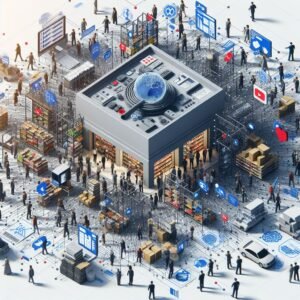
Building a Scalable Data Analytics Pipeline
In today’s data-driven business landscape, processing and analyzing large volumes of data efficiently can determine an organization’s success. Building a scalable data analytics pipeline is critical for businesses seeking to
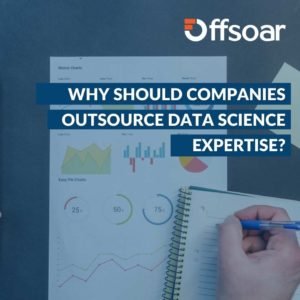
Why Should Companies Outsource Data Science Expertise?
Let’s address the elephant in the room right away. A business finds the greatest benefit in outsourcing talents because of one plain and simple reason.If you manage your data science in-house, you risk spending more money on hiring staff only to discover that there is insufficient work for them to accomplish or that your data science requirements differ from what you originally imagined.
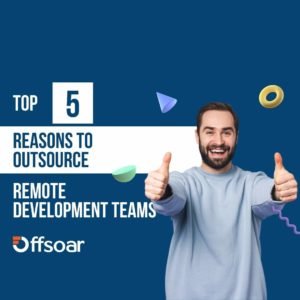
Top-5 reasons to Outsource Remote Development Teams
Remote work and outsourcing are here to stay, and the pandemic has proved this phenomenon further. It is of great benefit and bigger leverage to acquire and outsource brilliant technology