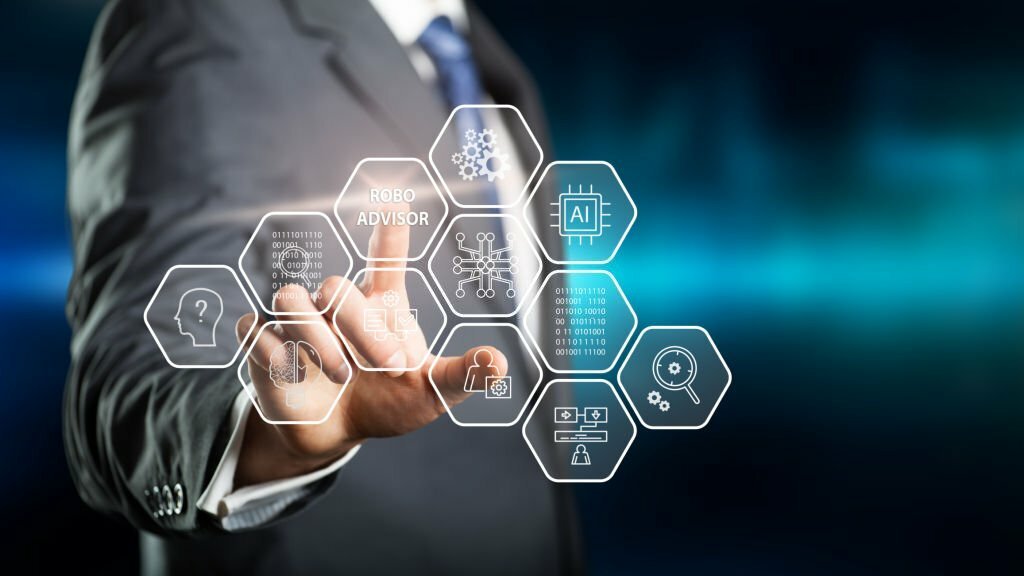
Data-Centric AI Development: Shifting the Focus from Model-Centric to Data-Centric AI
Recent years have witnessed remarkable advances in machine learning (ML) and artificial intelligence (AI), resulting in groundbreaking innovations in various sectors. Traditionally, developing intricate, highly optimised models has been the main goal of AI development.
Data-centric AI, an emerging trend, has shifted this approach nevertheless.
This change emphasises the importance of high-quality data more than model complexity.
This article looks at the key concepts of data-centric artificial intelligence (AI), as well as its benefits and revolutionary effects.
Understanding the Traditional Model-Centric Approach
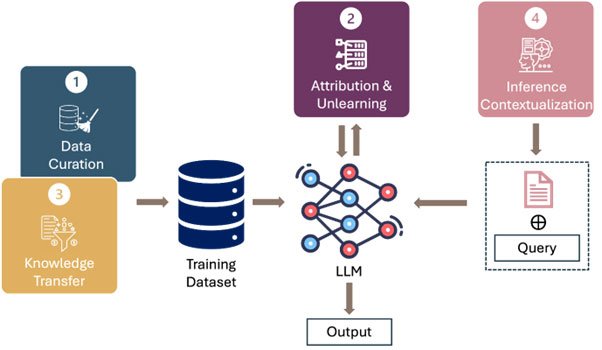
In its early stages, AI development focused mainly on model optimization. It was assumed that we might unlock improved performance by continuously improving model designs and using advanced algorithms.
This model-centric approach made new developments, such as deep learning networks and state-of-the-art algorithms, like GPT-3 and BERT, possible. With billions of parameters, these models are highly adept at performing complex tasks like natural language processing and recognizing images.
However, this approach’s shortcomings became apparent only quickly. Data quality often becomes a performance barrier, even with highly optimized models. Since large-scale models may only be as accurate and effective as the data they are trained on, noisy, biased, or inadequate data may significantly impact their performance.
Because of this, focus is increasingly being directed toward enhancing data quality, leading to the emergence of data-centric artificial intelligence.
What is Data-Centric AI?
Instead of continuously pushing for more complex models, data-centric AI focuses on enhancing the relevance, quality, and structure of the data used to train AI models.
In a data-centric paradigm, even simpler models can produce better outcomes with cleaner, more consistent, and representative datasets.
AI researchers, such as Andrew Ng, have contributed to popularising this trend by emphasising how improved data quality might lead to more valuable and scalable AI systems. The underlying principle is that “good data beats good models,” meaning that AI models may function better with more reliable data, even without significant modifications to their central architecture.
Key Components of Data-Centric AI
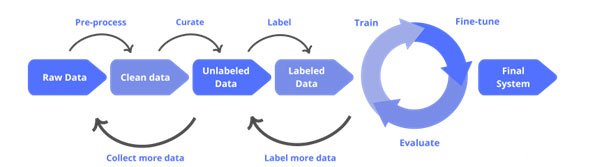
- Data Quality: The enhancement and refining of data are given top priority by data-centric AI. This comprises:
- Removing noise: Eliminating mistakes, inconsistencies, and outliers from datasets that may harm model performance.
- Handling Bias: Addressing dataset biases that may cause biased predictions or unethical AI behavior.
- Maintaining Consistency: Aligning methods for collecting data to ensure uniformity and proper training data structure.
- Data Labelling: Accurate data labeling is essential for supervised learning models to function correctly. Improving the precision and accuracy of labeled data is the main goal of data-centric artificial intelligence (AI), as this can significantly improve model performance. Methods like crowdsourcing and active learning are applied to enhance the quality of labeled datasets.
- Data Augmentation: Data-centric AI uses data augmentation techniques to artificially increase the size and variety of datasets. This is especially helpful when there is limited training data available. Techniques such as cropping, rotating, or applying filters may increase the model’s learning potential by creating variants of already-existing data points.
- Data Governance: Data governance systematically controls data quality throughout the data lifecycle. Data must be constantly reviewed, updated, and maintained for AI models to be reliable and scalable. A robust governance system ensures this.
Benefits of the Data-Centric Approach
- Improved Model Generalisation: Using a data-centric approach helps AI models be more generalizable. Clean, unbiased, and well-representative data gives models the best chance of operating correctly in a variety of real-world settings, producing more reliable outputs.
- Decreased Overfitting: Overfitting occurs when a model does well on training data but badly on unknown data. Models are less likely to overfit when they access high-quality, diverse datasets because they may learn more broadly applicable patterns rather than just memorizing specific instances.
- Faster Development Cycles: The AI development cycle may accelerate considerably when data refinement is prioritized above model complexity. Teams may focus on enhancing the quality and organization of data rather than testing ever more complex designs or fine-tuning hyperparameters. This may result in quicker iterations and deployments.
- Cost-effective AI Development: Training large, complex models requires a massive amount of computer resources. By putting data quality first, organizations may achieve greater performance with simpler models and save money on infrastructure and energy usage. This can also shorten training timeframes.
- Ethical & Responsible AI: Ensuring that models don’t reinforce preexisting biases or make unethical judgments is one of the biggest challenges in AI today. This problem is addressed by data-centric AI, which focuses on identifying and minimizing biases throughout the data preparation and collecting stages. AI systems may become more transparent, ethical, and fair in their decision-making processes by increasing the quality and diversity of their data.
Case Studies and Real-world Applications
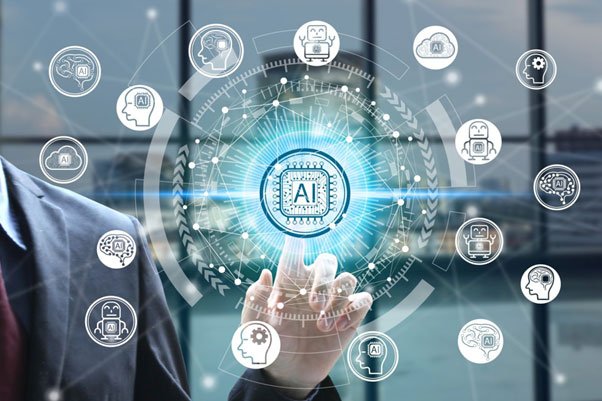
1. Healthcare
Clean, diverse datasets are essential for building diagnostic models in the field of healthcare. For example, research using AI-based cancer detection models found that models trained on representative, high-quality datasets performed better than those trained on bigger, noisier datasets.
Developers concentrating on enhancing data quality, such as ensuring a diverse set of patient demographics, made possible the creation of AI models with greater accuracy and generalisation across various patient groups.
2. Autonomous Vehicles
Manufacturers of autonomous vehicles mainly depend on the quality of training data. Companies such as Tesla and Waymo collect enormous volumes of driving data to train these models.
However, as the industry moves towards data-centric artificial intelligence, collecting edge-case data, such as rare but dangerous driving situations, becomes increasingly important.
Companies have witnessed advances in car safety and performance by refining the data rather than building increasingly complicated models.
3. Natural Language Processing (NLP)
A Data-Centric approach has been employed in NLP to improve the quality of textual data, hence fine-tuning models. Models may learn from a broader range of language patterns through data augmentation techniques like text expansion and paraphrasing. This improves performance in tasks like sentiment analysis and machine translation.
Conclusion
The future of AI development is poised to be data-driven rather than model-driven. Data-centric AI emphasizes the crucial importance of enhancing the quality, diversity, and structure of data to build AI systems that are more accurate, reliable, and ethically sound.
The understanding that “better data beats better models” is changing the AI landscape, even if model-centric innovation is still necessary for the field’s advancement.
By prioritizing data quality, organizations may fully utilize AI, resulting in faster development cycles, lower costs, and more reliable results.
Data is increasingly understood to be the real driver of AI success. Adopting data-centric principles will be essential as AI develops to create intelligent systems that are more efficient, ethical, and fairly constructed.
If you’re ready to embark on this journey and need expert guidance, subscribe to our newsletter for more tips and insights, or contact us at Offsoar to learn how we can help you build a scalable data analytics pipeline that drives business success. Let’s work together to turn data into actionable insights and create a brighter future for your organization.
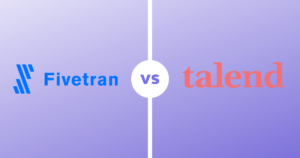
Top Data Integration Architecture Best Practices for Business Success
Best Practices for Data Integration Using Talend and Fivetran Through this article, we aim to highlight how data integration, merging data across many sources, is crucial in today’s modern data
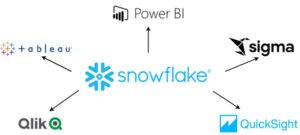
Snowflake Cloud Data Platform: Revolutionizing Data Warehousing in 2024
Snowflake: The Future of Cloud Data Warehousing for Scalable and Secure Data Management With its unmatched scalability, flexibility, and user-friendliness, Snowflake has become a prominent solution in cloud-based data warehousing. Although
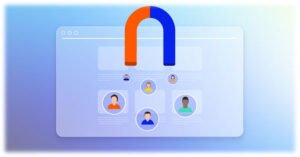
Addressing Customer Churn in SaaS: Effective Practices for Enhancing Retention and Sustained Growth
Leveraging CRM for Efficient User Management and Enhanced Customer Relationships Customer churn is a serious problem for software-as-a-service (SaaS) companies, where recurring revenue is essential to success. Churn reduces revenue
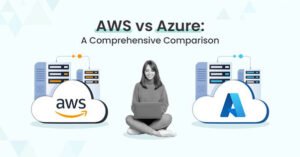
AWS vs Azure: A Comprehensive Comparison of Cloud Services for Data Analytics 2024
As data analytics increasingly integrates into business strategies choosing the appropriate cloud platform is essential. The two biggest names in the cloud space, Microsoft Azure and Amazon Web Services (AWS)Â provide
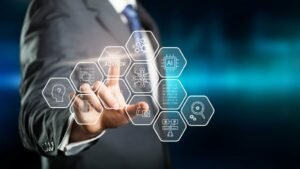
Data-Centric AI Development: Shifting the Focus from Model-Centric to Data-Centric AI
Recent years have witnessed remarkable advances in machine learning (ML) and artificial intelligence (AI), resulting in groundbreaking innovations in various sectors. Traditionally, developing intricate, highly optimised models has been the
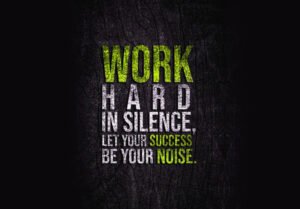
Mastering the Art of Quiet Success: Why Working in Silence Leads to Powerful Results in a Noisy World
In an era dominated by social media, where every moment of every day seems to be documented, curated, and shared, the phrase “Work in silence, let your success make the