Tackling AI Bias: Principles for Ethical AI Development and Fairness
AI Ethics and Bias Mitigation: Building Fair, Transparent, and Unbiased AI Systems
AI’s market value is expected to reach $407 billion by 2027, rising at 36% annually as more sectors embrace AI solutions. Since AI systems are increasingly used in recruiting, lending, and law enforcement, their tendency to reinforce human biases is a major worry. Nearly 45% of AI experts consider bias a major challenge in their projects, especially in sensitive areas. AI bias can cause unfair employment, lending, and law enforcement and worsen socioeconomic inequalities.
In domains where judgments directly affect people’s lives, fairness, openness, and bias reduction are critical for responsible AI development. We’ll discuss ethical AI system design to ensure technology enables equity rather than division.
Understanding AI Bias and Its Sources
Three often occurring reasons for artificial intelligence bias are:
Data Bias: Data bias is the result of imbalanced historical data or absent representation of several groups. A recruiting algorithm based primarily on prior successful workers of a corporation, for example, can favor some groups while excluding talented applicants from diverse backgrounds.
Algorithmic Bias: Algorithmic bias can be induced by the design of the algorithm even in cases when the data itself is balanced. Some models give particular traits that inadvertently help some groups top priority.
Societal Influence: Many artificial intelligence systems unwittingly mirror human biases seen in society. If trained on unprocessed internet data, an artificial intelligence language model can, for instance, create stereotyped depictions for particular demographics.
Knowing these sources of bias enables data scientists and developers to make better design choices to decrease inadvertent bias.
Why Fair and Unbiased AI Counts in Important Areas
Hiring: Resumes are screened, candidates are filtered, and even interviews are done using hiring algorithms. These methods can occasionally, however, reflect biases in the workforce. For example, it was discovered that Amazon’s AI hiring tool favored resumes with a male predominance, hence biased against women. Biassed recruiting algorithms can limit diversity and result in discriminating policies.
Lending: Artificial intelligence is also used to evaluate loan qualification. Should an artificial intelligence system discover from past data that underprivileged areas have greater default rates, it may grade individuals from those areas worse without regard for individual circumstances. By depriving equitable access to finance, this accentuates social inequalities and helps to prolong poverty cycles.
Law Enforcement: Law enforcement uses artificial intelligence in fields including risk analysis for parole decisions and predictive policing. These systems, meantime, are quite vulnerable to biased data. For example, a predictive policing program employed in several American cities was shown to unfairly target neighborhoods of color, therefore producing unfair results and further scrutiny. Protecting individuals’ rights and preventing unjust criminal justice procedures depend on fair artificial intelligence methods applied in law enforcement.
Principles for Ethical AI Development
Fairness: Fairness in artificial intelligence refers to the treatment of every person equally devoid of favoritism or discrimination against particular groups. By precisely balancing datasets and selecting neutral and pertinent model elements, artificial intelligence may be developed to be fair. This helps to stop specific groups from being methodically deprived.
Transparency: Public faith in artificial intelligence depends on transparency. Transparent artificial intelligence systems let users and stakeholders know why particular decisions are taken. Explainable artificial intelligence (XAI) and other methods let non-experienced people understand complicated models. Transparency is even more crucial in sensitive sectors to guarantee that artificial intelligence decisions may be assessed and rectified as required.
Accountability: Organizations and inventors of artificial intelligence should answer for the choices AI systems produce. This might imply keeping transparent about AI performance indicators, recording the process of AI development, and doing frequent audits. Establishing a culture of responsibility guarantees that, should they be found, prejudices are quickly and sensibly addressed.
Bias Mitigation Techniques in AI
Data Auditing: Frequent data audits help to spot training dataset biases. Datasets may be reviewed by developers to guarantee varied demographics are represented. For minority groups particularly, they might also look for data gaps that can compromise performance. Tools for analyzing datasets for fairness include IBM’s AI Fairness 360 (AIF360).
Algorithmic Adjustments: Some artificial intelligence systems allow one to minimize bias by means of algorithmic corrections. Fair models may be produced via re-weighting data, data balancing, and adversarial debiasing among other methods. For example, re-weighting data depending on underrepresented groups can help lower prejudice against these groups in employment processes.
Model Interpretability Tools: Transparency of AI models is facilitated by tools such as LIME (Local Interpretable Model-agnostic Explanations) and SHAP (Shapley Additive Explanations). They enable developers to see which elements affect AI decisions, therefore facilitating the detection of any prejudices. Especially in sensitive domains, these tools enable non-experts to grasp AI results and shed light on artificial intelligence decision-making.
The Role of Regulations and Standards
Conclusion
As AI becomes more integrated into our daily lives, justice, transparency, and accountability become increasingly important. Understanding the causes of bias, adhering to ethical standards, and using bias-reducing strategies can help us to design artificial intelligence systems supporting justice and equality all around. Ensuring that artificial intelligence benefits everyone, not just a few falls on developers, legislators, and companies. Once ethical artificial intelligence becomes the norm, we may create a future whereby technology advances society as a whole.
If you’re ready to embark on this journey and need expert guidance, subscribe to our newsletter for more tips and insights, or contact us at Offsoar to learn how we can help you build a scalable data analytics pipeline that drives business success. Let’s work together to turn data into actionable insights and create a brighter future for your organization.
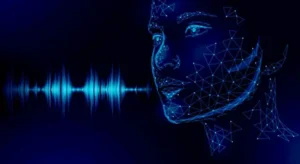
How LLMs Are Revolutionizing Text Mining and Data Extraction from Unstructured Data
Leveraging LLMs for Advanced Text Mining and Data Extraction from Unstructured Data Since digital transformation is growing exponentially, businesses generate huge amounts of unstructured data from sources like emails, PDFs,
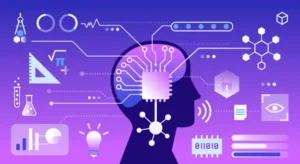
How Businesses Use LLMs for Competitive Intelligence to Stay Ahead of the Curve
How Businesses Use LLM’s for Data-Driven Competitive Intelligence to stay ahead of the curve Competitive intelligence (CI) is essential for keeping a competitive edge in today’s fast-paced business world. Businesses
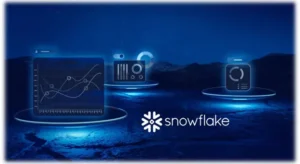
Maximizing Cost-Efficient Performance: Best Practices for Scaling Data Warehouses in Snowflake
Maximizing Cost-Efficient Performance: Best Practices for Scaling Data Warehouses in Snowflake Organizations rely on comprehensive data warehouse solutions to manage substantial volumes of data while ensuring efficiency and scalability. Snowflake,
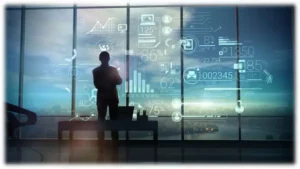
Comprehensive Guide to Implementing Effective Data Governance in Snowflake
Mastering Data Governance with Snowflake: A Comprehensive Guide Data governance is a systematic way to manage, organize, and control data assets inside an organization. This includes developing norms and policies
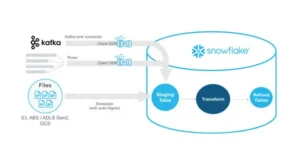
Efficiently Managing Dynamic Tables in Snowflake for Real-Time Data and Low-Latency Analytics
Managing Dynamic Tables in Snowflake: Handling Real-Time Data Updates and Low-Latency Analytics In this data-driven environment, businesses aim to use the potential of real-time information. Snowflake’s dynamic tables stand out
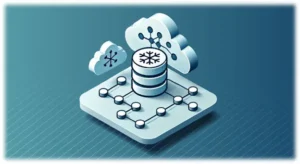
Mastering Data Lineage and Traceability in Snowflake for Better Compliance and Data Quality
Mastering Data Lineage and Traceability in Snowflake for Better Compliance and Data Quality In data-driven businesses, comprehending the source, flow, and alterations of data is essential. Data lineage is essential