AI-Powered Predictive Maintenance: Transforming Industry Efficiency and Equipment Lifespan
AI for Predictive Maintenance: Revolutionizing Manufacturing and Beyond
Understanding Predictive Maintenance (PDM)
How AI and Data Analytics Enable Predictive Maintenance
Key Components of AI-Driven Predictive Maintenance
Using artificial intelligence-driven predictive maintenance involves a few key components:
Data Collection and Processing
PDM begins with IoT sensors and devices continually monitoring machinery and sending data to central systems. Continuously gathering data on equipment conditions, these sensors detect temperature, pressure, and vibration. Often used for storage and processing this data are cloud-based solutions, which guarantee scalability and simple access for analysis.
Machine Learning Models
Artificial intelligence uses machine learning (ML) algorithms to forecast equipment failure. These algorithms are taught utilizing past data to identify particular trends pointing to possible problems. Anomaly detection models, for example, can highlight anomalies in machine performance or highlighting discrepancies pointing to wear or failure. Especially when tracking equipment with complicated operational patterns, neural networks, and time-series analysis are also somewhat frequently utilized.
Data Analysis and Decision-Making
AI’s true potential is in enabling the analysis to be taken action after the data is analyzed. It produces predictive insights and alarms, therefore enabling maintenance teams to respond proactively. For instance, a maintenance crew can act before the machine fails if artificial intelligence identifies an increase in vibration levels indicating possible bearing wear. Such realizations help flexible, just-in-time treatments instead of depending on scheduled maintenance cycles.
Benefits for Industries like Manufacturing
Predictive maintenance powered by artificial intelligence offers significant advantages in sectors where operational efficiency is a top priority:
- Reduced Downtime: PDM helps reduce unplanned breakdowns by estimating when equipment is most likely to fail. In an industry, where unscheduled downtime may stop lines of production and affect delivery dates, this is vital.
- Cost Savings: Reducing unneeded preventative maintenance schedules and lowering unscheduled repairs helps to minimize maintenance expenses. PDM helps businesses save costs by letting them concentrate resources where they are required. For instance, Airbus said that using predictive maintenance solutions powered by artificial intelligence resulted in a 20% drop in maintenance expenses.
- Extended Equipment Lifespan: AI’s exact observations of equipment condition enable prompt repair based on which wear and tear is prevented from developing into irreversible harm. This preventative maintenance helps machines last longer over time, therefore postponing the need for expensive replacements.
Challenges and Considerations
Data Quality and Quantity: High-quality data is mostly relied upon by artificial intelligence models to provide reliable forecasts. Low-quality or inconsistent data might produce inaccurate forecasts, therefore compromising the efficacy of the system.
Implementation Costs: Setting up AI-powered PDM solutions calls for IoT infrastructure, data storage, and qualified people investments. Though the long-term benefits are significant, smaller businesses may find the early expenses exorbitant.
Skills Gap: AI-driven PDM depends on knowledgeable staff to manage, understand, and polish data insights. Businesses might have to make training investments or recruit experts able to make good use of the data AI offers.
Conclusion
If you’re ready to embark on this journey and need expert guidance, subscribe to our newsletter for more tips and insights, or contact us at Offsoar to learn how we can help you build a scalable data analytics pipeline that drives business success. Let’s work together to turn data into actionable insights and create a brighter future for your organization.
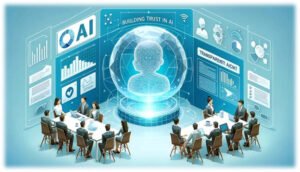
The Business Benefits of AI Transparency: Building Trust, Loyalty, and Profitability
AI Transparency Business Case: Why Open AI Models Are Good for Your Bottom Line Artificial intelligence is no longer a future idea; it is now the foundation of businesses ranging
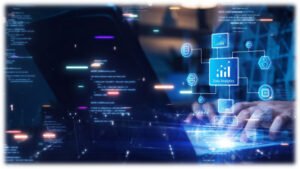
Harnessing LLMs for Real-Time Data Analysis and Smarter Decision-Making in Business
Harnessing the Power of LLMs for Real-Time Data Analysis and Decision-Making Across Industries Artificial Intelligence’s (AI) rapid growth can bring about transformations in many industries. Large language models (LLMs) like
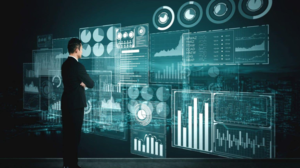
How Large Language Models (LLMs) Are Revolutionizing Business Intelligence
Transforming Business Intelligence with Large Language Models (LLMs) Data has emerged as a vital component of businesses in the digital transformation era. However, the sheer volume of unstructured data poses
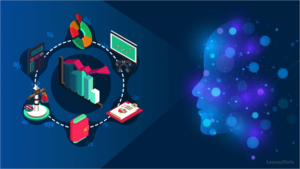
How LLMs Are Revolutionizing Customer Segmentation and Personalization in Data Analytics
How LLMs are Revolutionizing Customer Segmentation and Personalization in Data Analytics Businesses in the digital age struggle with managing the vast quantity of structured and unstructured data generated daily. Businesses
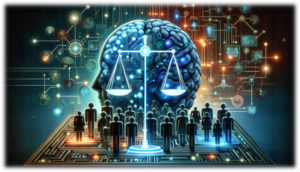
Tackling AI Bias: Principles for Ethical AI Development and Fairness
AI Ethics and Bias Mitigation: Building Fair, Transparent, and Unbiased AI Systems AI’s market value is expected to reach $407 billion by 2027, rising at 36% annually as more sectors
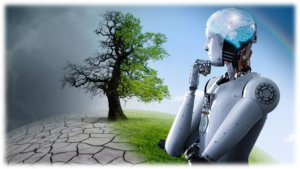
AI: Revolutionizing Environmental Sustainability and Business Practices for a Greener Future
AI and Environmental Sustainability: Tackling Climate Change, Optimizing Resources, and Promoting Sustainable Business Practices AI has undergone considerable evolution in many industries due to its effectiveness in its promotion of