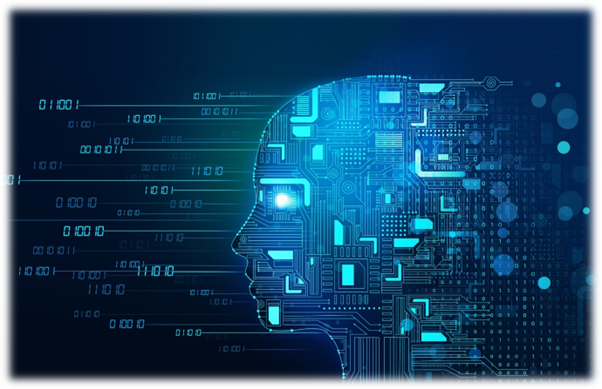
Quantum AI: Revolutionizing the Future of Artificial Intelligence with Quantum Computing
The Future of AI is Here: Quantum Computing Meets Artificial Intelligence!
Understanding Quantum Computing
AI and its current Limitation
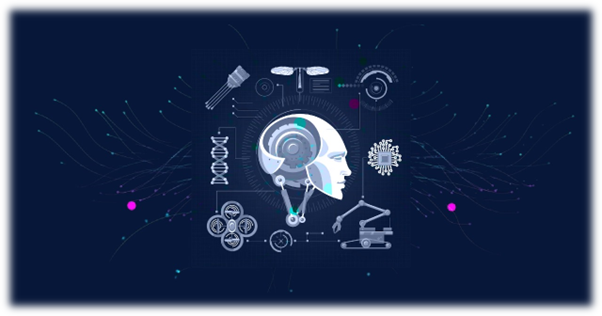
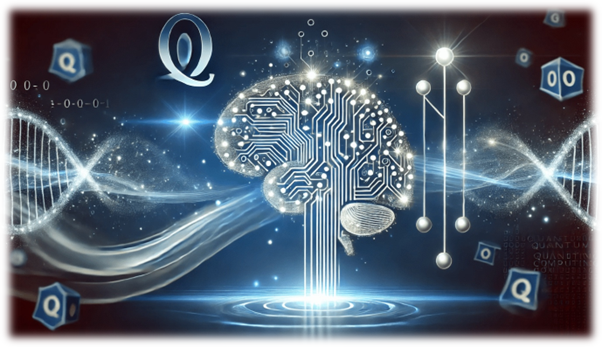
The intersection of Quantum Computing and AI
Revolutionizing Data Processing
Solving complex optimization problems
Enhancing Predictive Analysis
Challenges and the Path Forward
Conclusion
If you’re ready to embark on this journey and need expert guidance, subscribe to our newsletter for more tips and insights, or contact us at Offsoar to learn how we can help you build a scalable data analytics pipeline that drives business success. Let’s work together to turn data into actionable insights and create a brighter future for your organization.
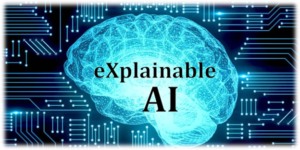
Explainable AI (XAI): Building Trust and Transparency in Artificial Intelligence
Explainable AI (XAI): Why Transparency in AI Models is More Important Than Ever In a world dominated by algorithms and machine learning, the mysterious inner workings of Artificial Intelligence (AI)
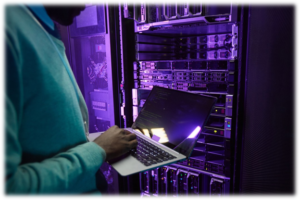
Generative AI and Synthetic Data: Revolutionizing Data Privacy and AI Model Training
Generative AI in Data Synthesis: Addressing Data Privacy and Enhancing Model Training Data has evolved in the digital age of today into the new money. It runs everything from companies
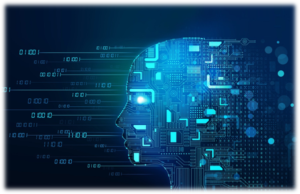
Quantum AI: Revolutionizing the Future of Artificial Intelligence with Quantum Computing
The Future of AI is Here: Quantum Computing Meets Artificial Intelligence! Artificial intelligence (AI) has revolutionized several areas of technology, including healthcare and finance. However, as AI applications become more
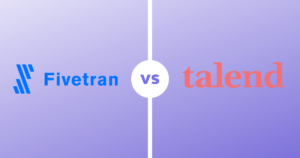
Top Data Integration Architecture Best Practices for Business Success
Best Practices for Data Integration Using Talend and Fivetran Through this article, we aim to highlight how data integration, merging data across many sources, is crucial in today’s modern data
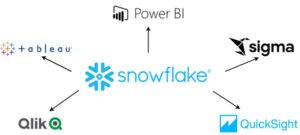
Snowflake Cloud Data Platform: Revolutionizing Data Warehousing in 2024
Snowflake: The Future of Cloud Data Warehousing for Scalable and Secure Data Management With its unmatched scalability, flexibility, and user-friendliness, Snowflake has become a prominent solution in cloud-based data warehousing. Although
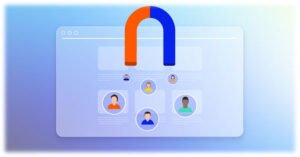
Addressing Customer Churn in SaaS: Effective Practices for Enhancing Retention and Sustained Growth
Leveraging CRM for Efficient User Management and Enhanced Customer Relationships Customer churn is a serious problem for software-as-a-service (SaaS) companies, where recurring revenue is essential to success. Churn reduces revenue