Managing Slowly Changing Dimensions (SCDs) in Snowflake for Data Integrity and Historical Analysis
Managing Slowly Changing Dimensions (SCDs) in Snowflake: Best Practices for Effective Data Modeling
Understanding Slowly Changing Dimensions (SCDs)
Types of SCDs
item_id | name | price | aisle |
93201 | Roasted Snacks | 3.99 | 11 |
07879 | Soft Drink | 7.99 | 13 |
If Roasted Snacks shift to aisle 6, SCD type 1 will reflect this change in the dimension table:
item_id | name | price | aisle |
93201 | Roasted Snacks | 3.99 | 6 |
07879 | Soft Drink | 7.99 | 13 |
SCD type 1 assures no duplicate records in the database and the latest current dimension. Real-time dashboarding and predictive modeling, when only the present situation matters, benefit from this.
Data practitioners cannot compare dimension changes over time since the table only stores the most current data. A data analyst would need more information to determine Roasted Snacks’ revenue increase after moving to aisle 6.
SCD type 1 simplifies current status reporting and analytics but limits history analysis.
SCD Type 2
While a table showing merely the present state is valuable, tracking previous changes to a dimension may be convenient and even necessary. SCD type 2 maintains historical data by creating a new row when a dimension changes and labeling it current and historical.
Simple, but implementation may be unclear. Look at an example.
This table is identical to the one we used for SCD type 1. However, a column was added. If the record has the most current value, is_current is true; otherwise, it is false.
item_id | name | price | aisle | is_current |
93201 | Roasted Snacks | 3.99 | 11 | True |
07879 | Soft Drink | 7.99 | 13 | True |
item_id | name | price | aisle | is_current |
93201 | Roasted Snacks | 3.99 | 11 | False |
07879 | Soft Drink | 7.99 | 13 | True |
93201 | Roasted Snacks | 3.99 | 6 | True |
Roasted Snacks’ new location is reflected in a new row with True in the is_current column. The prior record’s is_current column is set to False to preserve history data and appropriately portray current condition. Type 1 SCD
What if you wanted to see how Roasted Snack sales changed with location? If an object has several history records, utilizing a single column makes this impossible. This is easy, thankfully.
item_id | name | price | aisle | start_date | end_date |
93201 | Roasted Snacks | 3.99 | 11 | 2023-11-13 | 2099-12-31 |
07879 | Soft Drink | 7.99 | 13 | 2023-08-24 | 2099-12-31 |
The table would change if Roasted Snacks relocated to aisle 6 on January 4, 2024:
item_id | name | price | aisle | start_date | end_date |
93201 | Roasted Snacks | 3.99 | 6 | 2024-01-04 | 2099-12-31 |
07879 | Soft Drink | 7.99 | 13 | 2023-08-24 | 2099-12-31 |
93201 | Roasted Snacks | 3.99 | 11 | 2023-11-13 | 2024-01-03 |
The first row’s end_date is now the final day Roasted Snacks were in aisle 11. New record: Roasted Snacks in aisle 6. Start and end dates indicate when changes were made and which record is current.
This method for implementing SCD type 1 retains prior data and shows data changes. This let’s data analysts and scientists test operational changes, A/B test, and make educated decisions.
SCD Type 3
SCD type 3 is suitable for data that changes once or simply the most recent historical record. SCD type 3 stores changes in columns rather than “upserting” them as rows. This is difficult to explain, so let’s start with an example.
The table below lists US team sports. The table has two columns for current and past stadium names. Each club uses the original stadium name, hence the previous_stadium_name field has NULLs.
team_id | team_name | sport | current_stadium_name | previous_stadium_name |
562819 | Lafayette Hawks | Football | Triple X Stadium | NULL |
930193 | Fort Niagara Squirrels | Soccer | Musket Stadium | NULL |
team_id | team_name | sport | current_stadium_name | previous_stadium_name |
562819 | Lafayette Hawks | Football | Wabash Field | Triple X Stadium |
930193 | Fort Niagara Squirrels | Soccer | Musket Stadium | NULL |
Implementation
As shown in the diagram, we load data into the transactions database and collect change data using streams. We use the streams to generate landing tables, which offer the data structure for merging into D_PRODUCTs.
Step 1: Create data model table
console.log( 'Code is Poetry' );
CREATE OR REPLACE TABLE d_products (
product_code INTEGER NOT NULL PRIMARY KEY --type 0
,product_name VARCHAR(100) -- type 1
,product_price NUMBER(38,2) --type 2
,record_start_datetime TIMESTAMP_NTZ(9)
,record_end_datetime TIMESTAMP_NTZ(9)
);
Step 2: Create landing data tables
CREATE OR REPLACE TABLE transactions_landing (
transaction_number NUMBER(38,0),
customer_id NUMBER(38,0),
product_code NUMBER(38,0),
product_name VARCHAR(100),
transaction_datetime TIMESTAMP_NTZ(9),
units NUMBER(38,0),
value NUMBER(38,2),
price NUMBER(38,2),
shipping_address VARCHAR(1000)
);
CREATE OR REPLACE TABLE product_landing_scd_1(
product_code INTEGER
,product_name VARCHAR(100)
);
CREATE OR REPLACE TABLE product_landing_scd_2(
product_code INTEGER
,product_price NUMBER(38,2)
,transaction_datetime TIMESTAMP_NTZ(9)
);
Step 3: Track landing data table updates using streams.
We capture transaction landing table change data in streams in this stage.
CREATE OR REPLACE STREAM product_stream_scd_1 on table transactions_landing;
CREATE OR REPLACE STREAM product_stream_scd_2 on table transactions_landing;
Step 4: Copy source data to landing tables
Step 4 finishes setting up our tables and streams and imports staged data into TRANSACTIONS_LANDING.
COPY INTO transactions_landing from @transaction_data
FILES = ('WEBSITE_TRANSACTIONS_1.csv')
FILE_FORMAT = (TYPE = CSV, SKIP_HEADER = 1);
Step 5: SCD Data Processing
Since data is in the TRANSACTIONS_LANDING database and our streams record change data, we can process SCD data with the MERGE command.
First, we’ll use a merge statement to retrieve data from the SCD 2 stream and insert new product prices to meet our type 2 row history.
MERGE INTO product_landing_scd_2 prod_land_2 USING
(SELECT
product_code
,price
,MAX(transaction_datetime) as transaction_datetime
FROM product_stream_scd_2
GROUP BY product_code,price) prod_strm
ON prod_land_2.product_code = prod_strm.product_code
AND prod_land_2.product_price = prod_strm.price
WHEN NOT MATCHED THEN INSERT
(product_code
,product_price
,transaction_datetime)
VALUES
(prod_strm.product_code
,prod_strm.price
,prod_strm.transaction_datetime);
Next, we develop a product name lookup database to update product names depending on product code and last transaction.
MERGE INTO product_landing_scd_1 prod_land_1 USING
(SELECT DISTINCT
product_stream_scd_1.transaction_number
,product_code
,pn.product_name
FROM product_stream_scd_1
JOIN (SELECT product_name, max(transaction_number) as max_transaction from product_stream_scd_1 group by product_name) pn
ON product_stream_scd_1.product_name = pn.product_name
AND product_stream_scd_1.transaction_number = pn.max_transaction) prod_strm
ON prod_land_1.product_code = prod_strm.product_code
WHEN MATCHED THEN UPDATE SET
prod_land_1.product_name = prod_strm.product_name
WHEN NOT MATCHED THEN INSERT
(product_code
,product_name)
VALUES
(prod_strm.product_code
,prod_strm.product_name);
We replace any outdated product names with the current one.
MERGE INTO d_products USING
(SELECT
product_code
,product_name
FROM product_landing_scd_1) prod_land
ON d_products.product_code = prod_land.product_code
WHEN MATCHED AND (d_products.product_name != prod_land.product_name) THEN UPDATE SET
d_products.product_name = prod_land.product_name;
Finally, we combine our product pricing landing table into D_PRODUCTS with the latest product name.
MERGE INTO d_products USING
(SELECT
p2.product_code
,product_name
,product_price
,transaction_datetime as record_start_datetime
,lead(transaction_datetime) OVER (PARTITION BY p2.product_code ORDER BY transaction_datetime) as record_end_datetime
FROM product_landing_scd_2 p2
JOIN product_landing_scd_1 p1 on p2.product_code = p1.product_code) prod_land
ON d_products.product_code = prod_land.product_code
AND d_products.product_price = prod_land.product_price
WHEN MATCHED THEN UPDATE SET
d_products.record_end_datetime = prod_land.record_end_datetime
WHEN NOT MATCHED THEN INSERT
(product_code
,product_name
,product_price
,record_start_datetime
,record_end_datetime)
VALUES
(prod_land.product_code
,prod_land.product_name
,prod_land.product_price
,prod_land.record_start_datetime
,prod_land.record_end_datetime);
After processing, the data is organized according to the data model. Product_Name and Price are SCD Types 1 and 2.
Advantages and Challenges
Best practices and Tips
Conclusion
If you’re ready to embark on this journey and need expert guidance, subscribe to our newsletter for more tips and insights, or contact us at Offsoar to learn how we can help you build a scalable data analytics pipeline that drives business success. Let’s work together to turn data into actionable insights and create a brighter future for your organization.
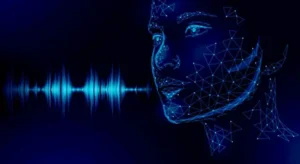
How LLMs Are Revolutionizing Text Mining and Data Extraction from Unstructured Data
Leveraging LLMs for Advanced Text Mining and Data Extraction from Unstructured Data Since digital transformation is growing exponentially, businesses generate huge amounts of unstructured data from sources like emails, PDFs,
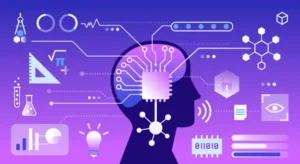
How Businesses Use LLMs for Competitive Intelligence to Stay Ahead of the Curve
How Businesses Use LLM’s for Data-Driven Competitive Intelligence to stay ahead of the curve Competitive intelligence (CI) is essential for keeping a competitive edge in today’s fast-paced business world. Businesses
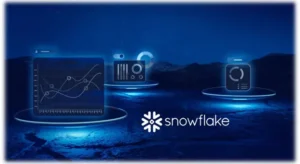
Maximizing Cost-Efficient Performance: Best Practices for Scaling Data Warehouses in Snowflake
Maximizing Cost-Efficient Performance: Best Practices for Scaling Data Warehouses in Snowflake Organizations rely on comprehensive data warehouse solutions to manage substantial volumes of data while ensuring efficiency and scalability. Snowflake,
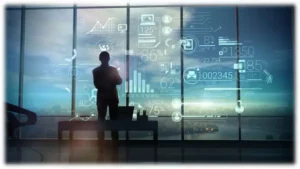
Comprehensive Guide to Implementing Effective Data Governance in Snowflake
Mastering Data Governance with Snowflake: A Comprehensive Guide Data governance is a systematic way to manage, organize, and control data assets inside an organization. This includes developing norms and policies
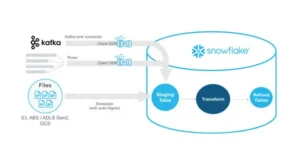
Efficiently Managing Dynamic Tables in Snowflake for Real-Time Data and Low-Latency Analytics
Managing Dynamic Tables in Snowflake: Handling Real-Time Data Updates and Low-Latency Analytics In this data-driven environment, businesses aim to use the potential of real-time information. Snowflake’s dynamic tables stand out
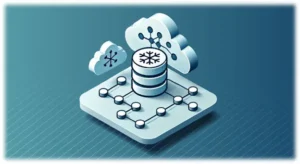
Mastering Data Lineage and Traceability in Snowflake for Better Compliance and Data Quality
Mastering Data Lineage and Traceability in Snowflake for Better Compliance and Data Quality In data-driven businesses, comprehending the source, flow, and alterations of data is essential. Data lineage is essential