The Business Benefits of AI Transparency: Building Trust, Loyalty, and Profitability
AI Transparency Business Case: Why Open AI Models Are Good for Your Bottom Line
The Increasing Importance of AI Transparency
Ethical and Regulatory Drivers
Consumer Expectations
Transparency is about individuals, not just regulators. Consumers are increasingly expecting businesses to justify the judgments their AI models make. According to an Accenture poll, 76% of customers trust organizations that freely explain how their AI systems work.
Business Advantages of Transparent AI Models
Building Customer Trust and Loyalty
Competitive Differentiation
Improves Decision-Making
Reducing Legal and Reputational Risks
How Transparent AI affects the bottom line
Revenue Growth
Clients who trust your AI are more inclined to use your services. For example, a retail giant that used explainable AI for tailored buying suggestions reported a 20% boost in repeat sales. Why? Customers enjoyed how clear and relevant the advice was.
Cost Reduction
Legal fights and public relations disasters are expensive. By making your AI models apparent, you may avoid these expenses. Furthermore, explainable AI facilitates audits and troubleshooting, saving time and money for compliance.
Employee Efficiency
Practical Steps for Creating Transparent AI Models
Prioritize explainability from the Start
Transparency should not be an afterthought. Choose explainability-focused algorithms and techniques, such as decision trees and interpretable neural networks. Frameworks such as SHAP and LIME can also assist in making complicated models easier to grasp.
Create a Culture of Transparency
Transparency begins with the people you hire. Encourage collaboration among data scientists, business executives, and ethical officers to ensure that everyone appreciates the value of explainability. Regular training and open discourse may promote an environment in which transparency is the norm.
Communicate Clearly With End Users
Customers do not need a PhD in data science to comprehend your AI. Invest in intuitive interfaces and explicit explanations for AI-powered judgments. For example, FinTech applications that give visual explanations for credit score adjustments have received increased customer satisfaction.
Real-world Success Stories
Healthcare: A hospital network uses explainable AI for patient triage. The transparent method enhanced decision accuracy while also reassuring patients and families, hence increasing confidence.
Retail: An e-commerce business deployed transparent AI for pricing algorithms, earning a reputation for fairness. Sales rose as clients gained confidence in their prices.
Finance: A bank used explainable credit scoring, which reduced disputes by 30% and improved regulatory compliance.
The Future of AI Transparency in Business
Conclusion
If you’re ready to embark on this journey and need expert guidance, subscribe to our newsletter for more tips and insights, or contact us at Offsoar to learn how we can help you build a scalable data analytics pipeline that drives business success. Let’s work together to turn data into actionable insights and create a brighter future for your organization.
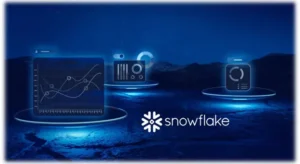
Maximizing Cost-Efficient Performance: Best Practices for Scaling Data Warehouses in Snowflake
Maximizing Cost-Efficient Performance: Best Practices for Scaling Data Warehouses in Snowflake Organizations rely on comprehensive data warehouse solutions to manage substantial volumes of data while ensuring efficiency and scalability. Snowflake,
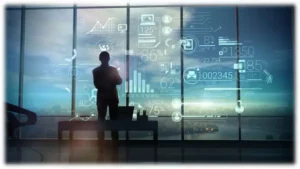
Comprehensive Guide to Implementing Effective Data Governance in Snowflake
Mastering Data Governance with Snowflake: A Comprehensive Guide Data governance is a systematic way to manage, organize, and control data assets inside an organization. This includes developing norms and policies
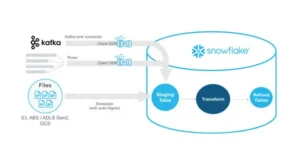
Efficiently Managing Dynamic Tables in Snowflake for Real-Time Data and Low-Latency Analytics
Managing Dynamic Tables in Snowflake: Handling Real-Time Data Updates and Low-Latency Analytics In this data-driven environment, businesses aim to use the potential of real-time information. Snowflake’s dynamic tables stand out
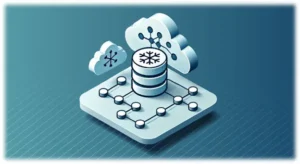
Mastering Data Lineage and Traceability in Snowflake for Better Compliance and Data Quality
Mastering Data Lineage and Traceability in Snowflake for Better Compliance and Data Quality In data-driven businesses, comprehending the source, flow, and alterations of data is essential. Data lineage is essential
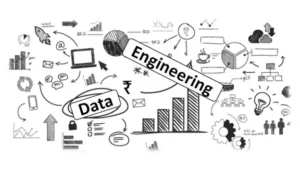
Revolutionizing Data Preparation with LLMs: Automating ETL Processes for Faster Insights
How LLMs Are Revolutionizing Data Preparation and ETL Processes for Better Insights Data preparation is the foundation of analytics, which serves as the link between raw data and useful insights.
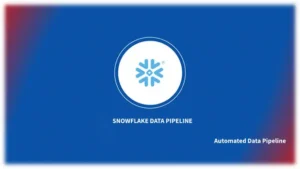
Best Practices for Building Reliable Snowflake Data Pipelines: Ensure Consistency and Performance
Building Reliable Snowflake Data Pipelines: Best Practices for Consistency and Performance Data pipelines serve as the foundation of contemporary analytics, facilitating decision-making by converting raw data into actionable insights. Snowflake,